Welcome to CADASIL
Prediction of the functional significance of missense variants of CADASIL syndrome
Analyse your variant
Know our pathogenicity predictors
To assess the functional significance of missense variants, we have developed a specific predictor for NOTCH3 protein. Predict your variant or check our examples to see the result
CADASIL
Prediction of the functional significance of missense variants affecting NOTCH3 protein involved in CADASIL syndrome, the most common form of hereditary stroke disorder
Disclaimer This resource is uniquely intended for research purposes. The authors are not responsible for neither its use nor misuse. The data provided are not intended as advice of any kind. The authors have worked with care in the development of this server, but assume no liability or responsibility for any error, weakness, incompleteness or temporariness of the resource and of the data provided.
How to interpret a prediction?
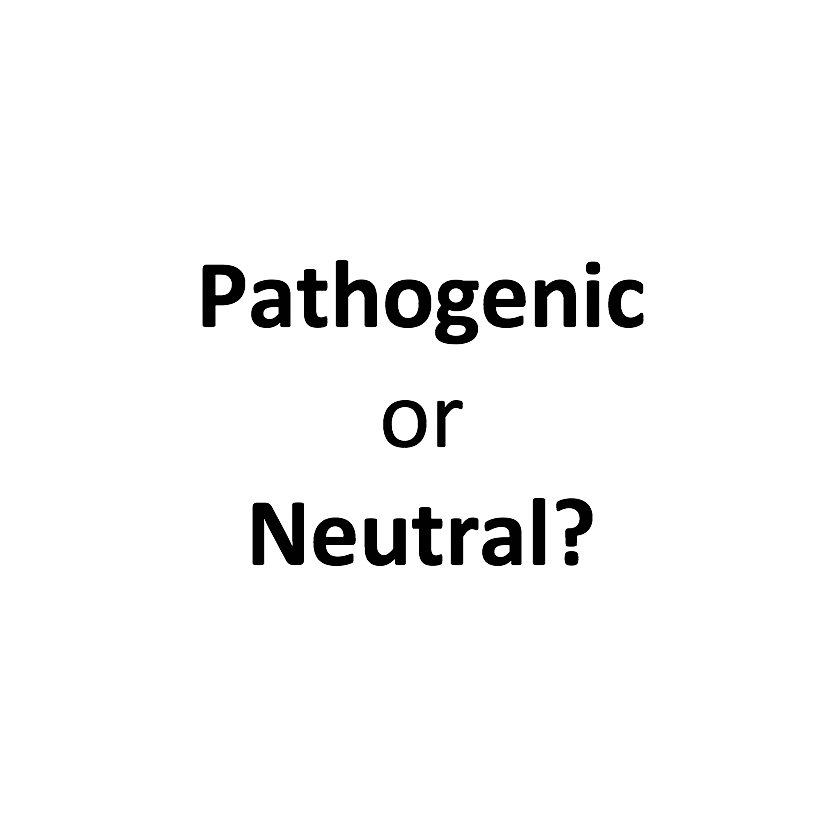
Prediction Label
The variants are classified as pathogenic or neutral by a supervised machine learning algorithm
The label depends in the numeric score. Variants above 0.5 are pathogenic while variants low 0.5 are neutral
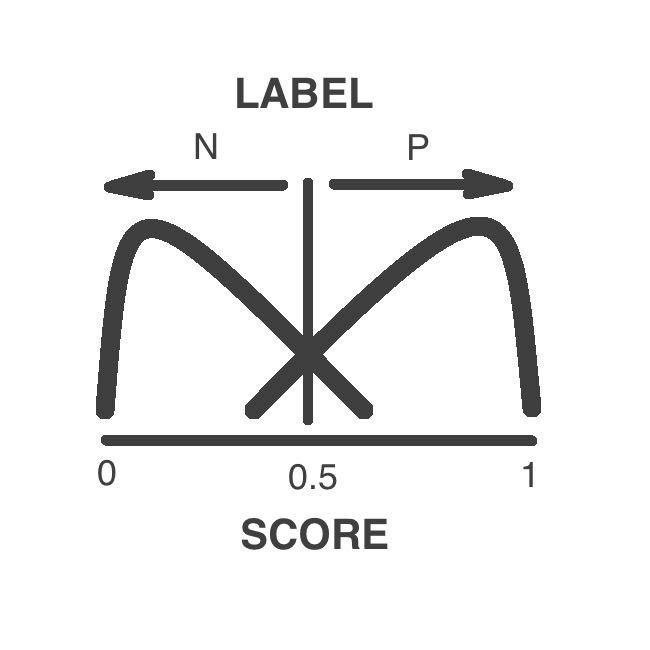
Prediction Score
Beyond the label, a variant is characterized with a numerical score of its pathogenicity. We provide you with this score, so you can have a more precise sense of the consequence of the variant
This score has a continuous range between 0 to 1, being 0 a neutral and 1 a pathogenic variant. The threshold between pathogenic and neutral variant is at 0.5
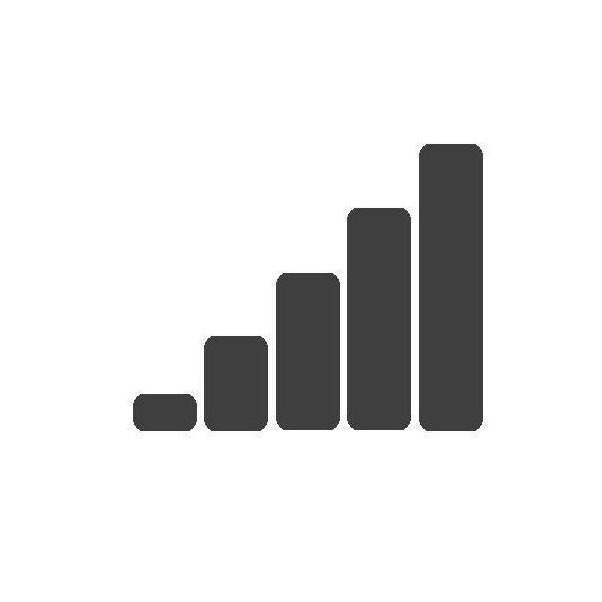
Prediction Reliability
Such as any other measurement, a predictions has an associated error. This metric measures the accuracy of the prediction
The reliability has a continuous range between 0 to 1, being 1 a trueful prediction
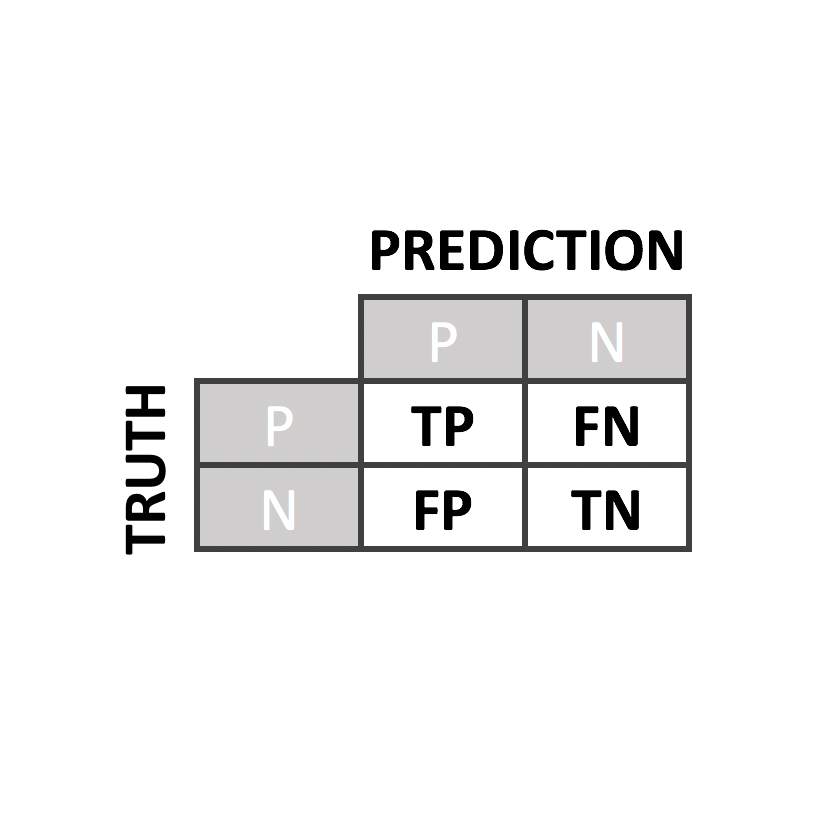
Predictor Performance
We have evaluated our predictors with rigorous quality controls and compared to the state of the art predictors such as PolyPhen2, PON-P2, SIFT or CADD
To evaluate our predictor, we perform a leave-one-out cross-validation. From it, we derived several performance metrics such as sensitivity, specificity, accuracy, AUC and MCC